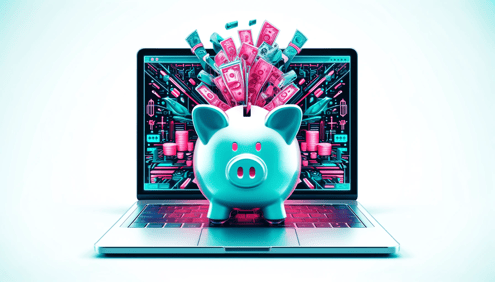
Show Me The Money: Building a Profitable eDiscovery Cost Recovery Model
In the beginning, there was paper and lots of it.
Think stacks of bankers' boxes big enough to make...
Read Story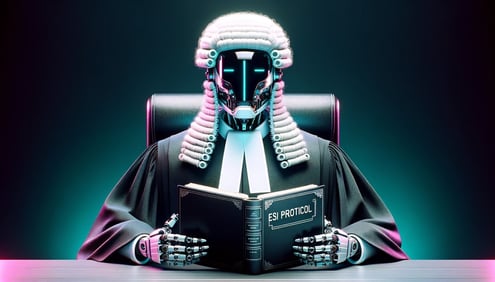
What is an ESI Protocol? And Why Should I Care?
In today's blog, we delve into the intricate world of ESI Protocols. This might have some...
Read Story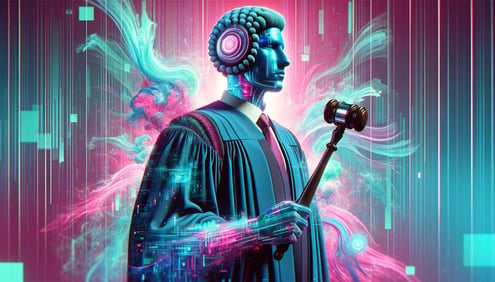
Beyond the Bench, 10 Unexpected Uses for eDiscovery Tools
When most people think of the term Electronic Discovery (eDiscovery), they think of hordes of...
Read Story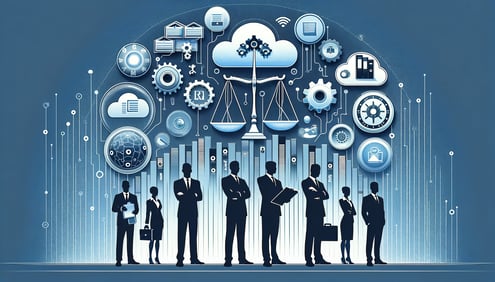
The Art of Records Requests: Tips, Tricks, and Insights from the Front Lines
In the digital era, where data proliferates at an unprecedented rate, the task of managing record...
Read Story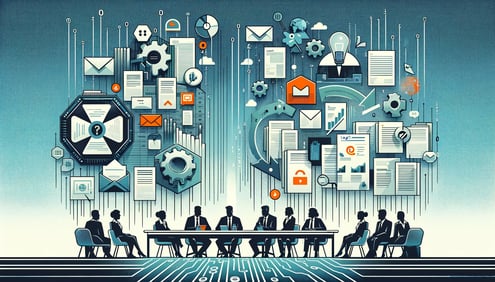
Frequently Asked Questions from “The Art of Records Requests: Tips, Tricks, and Insights from the Front Lines”
In the information age, public records management has become an increasingly complex and demanding...
Read Story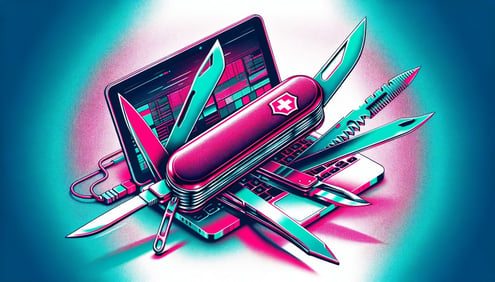
5 Ways eDiscovery Technology Makes You an Ultimate Cyber MacGyver
What does an 80’s heartthrob with a magical mullet and knack for turning duct tape and a piece of...
Read Story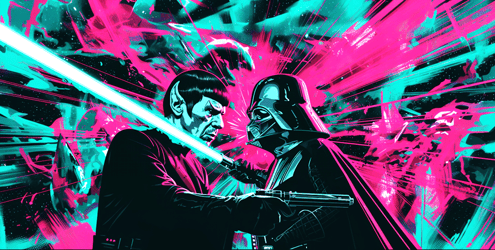
Phasers Or Lightsabers: Which Guides The Future Of eDiscovery
The Modern dataverse is a sprawling expanse filled with warp drives, hyperdrives, and the...
Read Story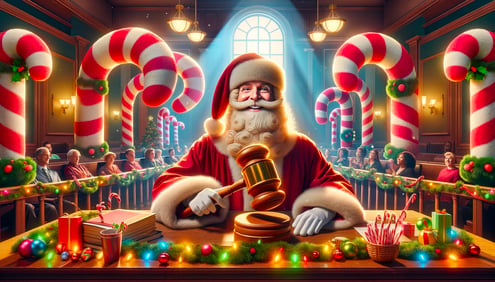
Candy Canes and Courtrooms: All-Time Best Holiday Movies For Lawyers
As the holiday season rolls in, it's the perfect time for lawyers, paralegals, and legal tech...
Read Story